

Efforts during the last decade have organized large amounts of diverse information as collections of nodes (entities) and edges (relationships) 12, 13, 14, 15, 16. Networks and graphs have emerged as natural ways of representing connected data, including also in biology 9, 10, 11.

Therefore, a knowledge-based platform that integrates a range of databases and scientific literature information with omics data into an easy-to-use workflow would empower discovery science and clinical practice. Moreover, we see an increasing need for more inclusive solutions that provide those with little expertise with tools for extracting high-quality information from proteomics data in a more user-friendly manner. Only a handful of tools have been aimed at alleviating this problem 7, 8 There is a need for solutions that integrate multiple data types while capturing the relationships between the molecular entities and the resulting disease phenotype. An even larger and growing bottleneck in high-throughput proteomics is the difficulty of interpreting the quantitative results to formulate biological or clinical hypotheses. However, currently used MS-based proteomics workflows were conceptualized more than a decade ago, and rapidly increasing data volumes are posing new challenges for the field. Over the last decade, mass spectrometry (MS)-based proteomics has advanced greatly and now provides an increasingly comprehensive view of biological processes, cellular signaling events and protein interplay 6.
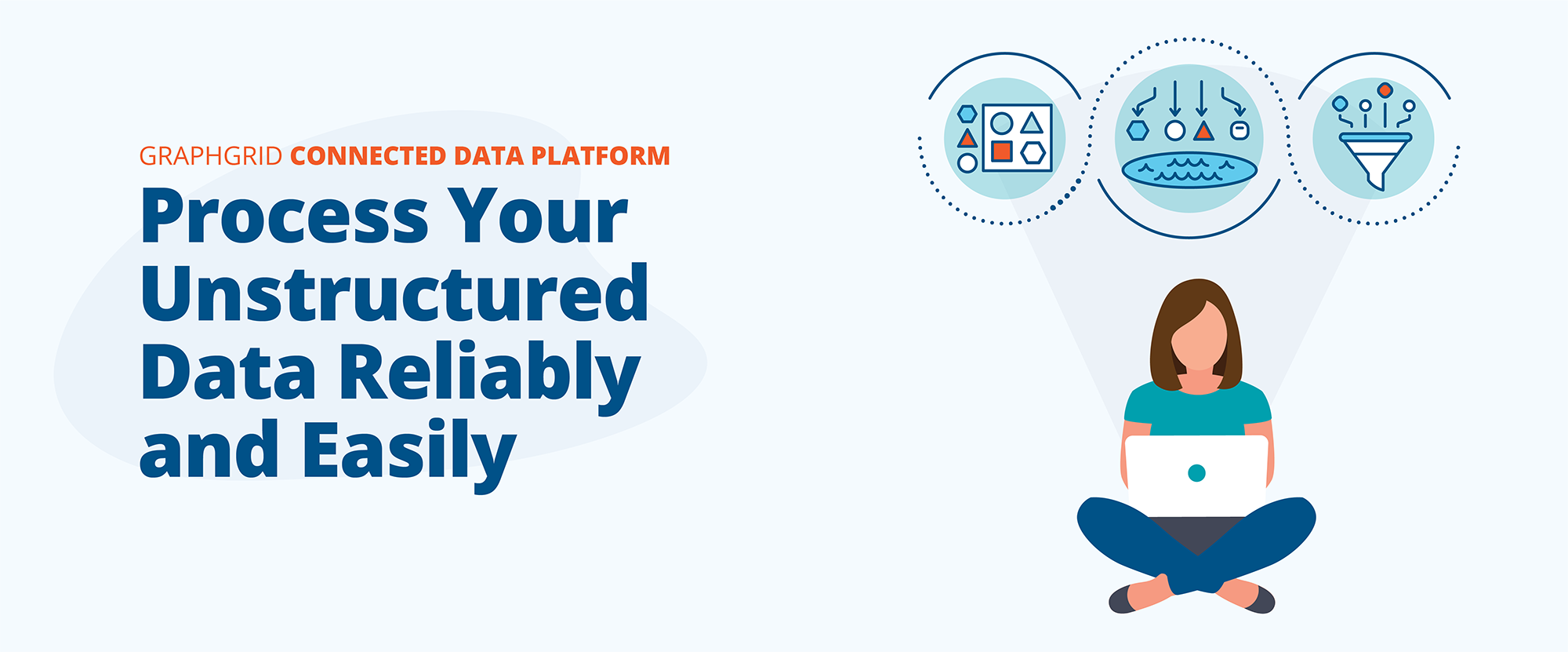
Moreover, much scientific data and knowledge are only ‘stored’ within millions of unstandardized journal publications. However, harmonization and integration is still challenging because it is often diverse, heterogeneous and distributed across multiple platforms. The biomedical research community has long recognized the need to collect, organize and structure the relevant data, resulting in community-wide adoption of multiple biomedical databases (Supplementary Table 1). Moreover, multiomics data can generate new hypotheses that ultimately translate into clinically actionable results 5. Recently, we found that a more fine-grained definition of disease that combines clinical and molecular data can provide a deeper understanding of individuals’ disease phenotypes and reveal candidate markers of prognosis and/or treatment 2, 3, 4. This requires seamless integration of diverse data, such as clinical, laboratory, imaging and multiomics data (genomics, transcriptomics, proteomics or metabolomics) 1. The paradigm of evidence-based precision medicine has evolved toward a more comprehensive analysis of disease phenotypes.
